Data is the lifeblood of modern organizations. It fuels decision-making, drives innovation, and enables businesses to stay competitive in today’s data-driven world. However, data’s sheer volume and complexity can be overwhelming without a proper data management strategy. If you’re interested in learning more about what is data management, this guide will explore its essential components and why it is crucial for businesses of all sizes.
What is data management and why is it important?
Data management involves collecting, organizing, analyzing, and storing data to ensure the accuracy, accessibility, and security of the system. It consists of implementing policies, procedures, and technologies to effectively manage data throughout its lifecycle.
Firstly, it ensures data quality. By establishing standards and processes for data collection and validation, organizations can minimize errors and ensure the reliability of their data. This, in turn, leads to better decision-making and improved operational efficiency.
Secondly, data management enables data integration. Organizations often deal with data from various sources and systems in today’s interconnected world. Businesses can combine disparate data sets by implementing data management practices, enabling a holistic view of their operations and facilitating data-driven insights.
Lastly, it is crucial for data security and compliance. With the increasing frequency of data breaches and privacy concerns, organizations must prioritize protecting sensitive data. By implementing robust practices, businesses can safely store their data from unauthorized access and ensure it works under relevant regulations.
The key components of data management
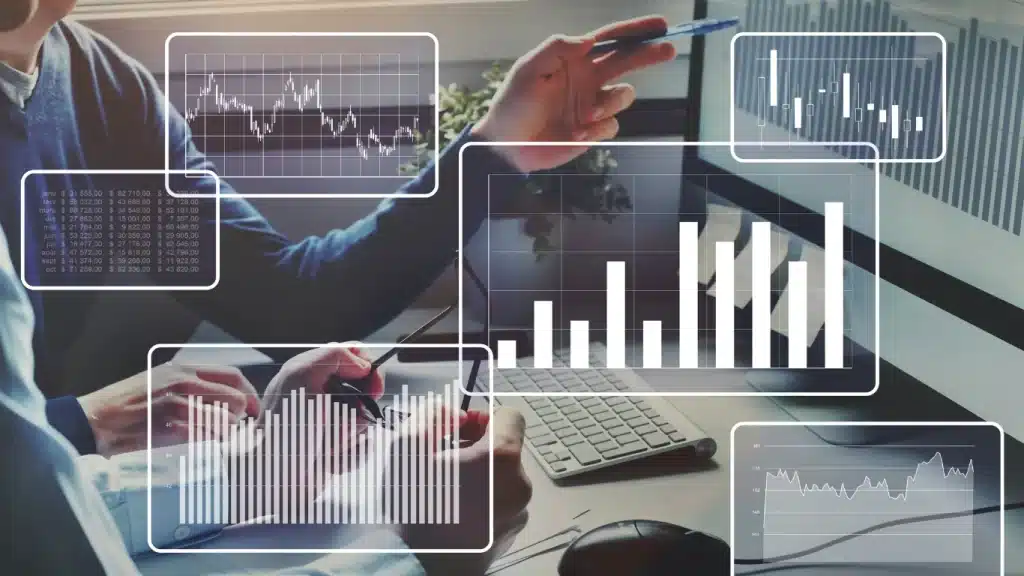
Data management encompasses various components that work together to ensure the effective handling of data. These components include –
- Data governance
- Data quality
- Data integration
- Data migration
- Data storage
- Data security
- Data analytics
- Data visualization
Data governance and data quality
Data governance and data quality are two critical components of effective data management. Governance establishes the framework for managing data assets, while data quality ensures data accuracy, consistency, and reliability.
Data governance involves defining policies, procedures, and organisational data management responsibilities. It establishes guidelines for data usage, privacy, security, and compliance. By implementing governance practices, organizations can ensure that data is accurate, consistent, and aligned with business objectives.
Data quality, on the other hand, focuses on data fitness for its intended purpose. It involves assessing and improving data accuracy, completeness, consistency, and timeliness. Data quality management practices include data cleansing, data profiling, and data validation to ensure data integrity and reliability.
Effective data governance and data quality management go hand in hand. Data governance frameworks provide the structure and guidelines for data quality management practices. By establishing governance policies and procedures, organizations can create a culture of data quality and ensure that data is appropriately managed throughout its lifecycle.
Data integration and data migration
Data integration and data migration are two essential processes in data management. Integration combines information from multiple sources into an overall view, while migration transfers data from one system or storage location to another.
Data integration enables organizations to gain insights from disparate data sets and make informed decisions. It involves data extraction, transformation, and loading (ETL) processes and real-time data integration through application programming interfaces (APIs). Organisations can view their operations by combining data from numerous sources and gaining valuable insights.
Data migration, on the other hand, is often necessary when organizations upgrade their systems or adopt new technologies. It requires careful planning, data mapping, and validation to ensure an easy transition without data loss or corruption. Migration projects involve extracting data from the source system, adjusting it to fit the target system’s requirements, and loading it into the new system.
Both data integration and migration require robust data management practices. Organizations must ensure that data transforms accurately and is securely transferred during these processes. Effective data integration and migration strategies help organizations support business growth and innovation by leveraging their data assets.
Data storage and data security
Data storage and data security are critical aspects of data management. Data storage involves data’s physical or virtual storage, while data security ensures data protection from unauthorized access, loss, or corruption.
Data storage technologies have evolved significantly, offering organisations various data storage options. Traditional options include on-premises data centres, while cloud-based storage solutions provide scalability, flexibility, and cost-efficiency. Organizations must assess data storage needs based on data volume, accessibility requirements, and security considerations.
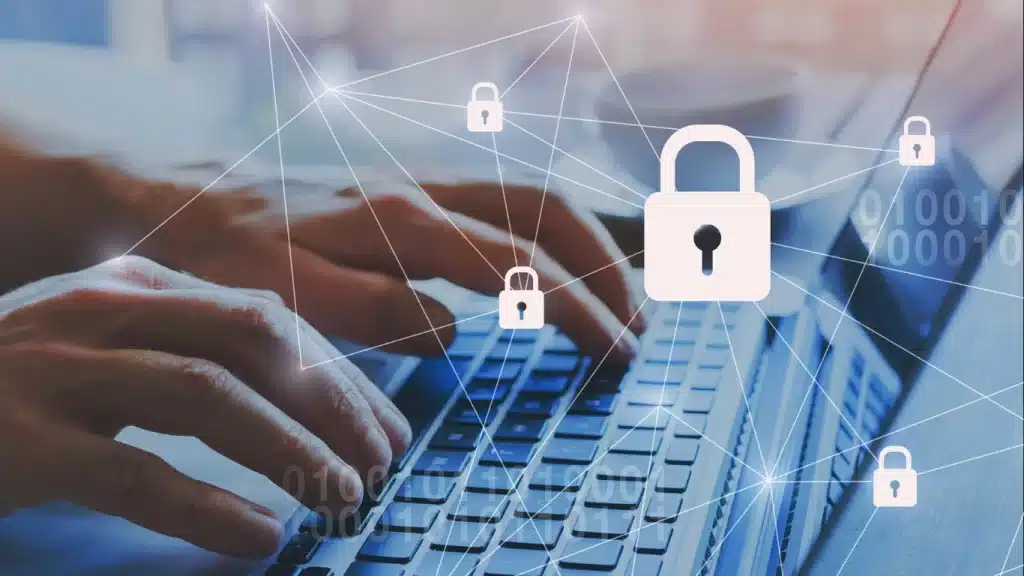
Given the high frequency and sophistication of cyber threats, data security is a top priority for organisations. Robust data security strategies involve implementing access controls, encryption, and data backup solutions. Organizations must comply with data protection regulations, such as GDPR.
Organizations can ensure their data assets’ availability, confidentiality, and integrity by implementing adequate data storage and security measures. This, in turn, enables them to build trust with customers, protect sensitive information, and mitigate the risks associated with data breaches.
Data analytics and data visualization
Data analytics and visualization are crucial components of data management that enable organizations to derive actionable insights from their data.
Data analytics involves using advanced techniques and algorithms to analyze large volumes of data and uncover patterns, trends, and correlations. It encompasses various approaches, including descriptive, diagnostic, predictive, and prescriptive analytics. Organizations can make data-driven decisions, optimize processes, and identify new business opportunities by leveraging data analytics.
Data visualization, on the other hand, focuses on presenting data visually, such as charts, graphs, and dashboards. Visual representations of data enable users to understand complex information and identify trends or outliers quickly. Data visualization tools provide interactive and intuitive interfaces for exploring data, allowing users to gain insights at a glance.
Both data analytics and data visualization rely on high-quality and well-managed data. Effective data management practices, such as data integration and quality management, are essential for accurate and meaningful analytics and visualization. By leveraging data analytics and visualization, organizations can unlock the potential of their data assets and informed decisions.
Types of Data Management
Operational Data Management: Operational data management is the process of managing data that is used to support daily business operations. It involves collecting, storing, and managing transactional data, such as customer orders, invoices, and inventory levels.
Analytical Data Management: Analytical data management manages data used for analytical purposes, such as data mining, predictive analytics, and business intelligence. It involves collecting, storing, and operating data to support decision-making and strategic planning.
Engineering Data Management: Engineering data management is the process of managing data used in product design, development, and manufacturing. It involves collecting, storing, and managing data related to product design, testing, and production.
Apprenticeships Data Management System: Apprenticeships data management system manages data used to support apprenticeship programs. It involves collecting, storing, and managing data related to apprenticeships, such as apprenticeship agreements, training plans, and progress reports.
How to effectively go about data management?
Effective data management requires a holistic approach encompassing all aspects of data handling, from collection to disposal. It involves the implementation of robust policies, procedures, and technologies to ensure the accuracy, accessibility, and security of data.
To achieve effective data management, organizations should –
- Develop a data management strategy: Define the objectives, scope, and priorities of data management efforts. Align the plan with business goals and objectives.
- Establish data governance frameworks: Define roles, responsibilities, and processes for data management. Establish standards and guidelines for data usage, privacy, and security.
- Implement data quality management practices: Assess and improve data accuracy, completeness, consistency, and timeliness. Ensure data integrity and reliability through data cleansing, profiling, and validation.
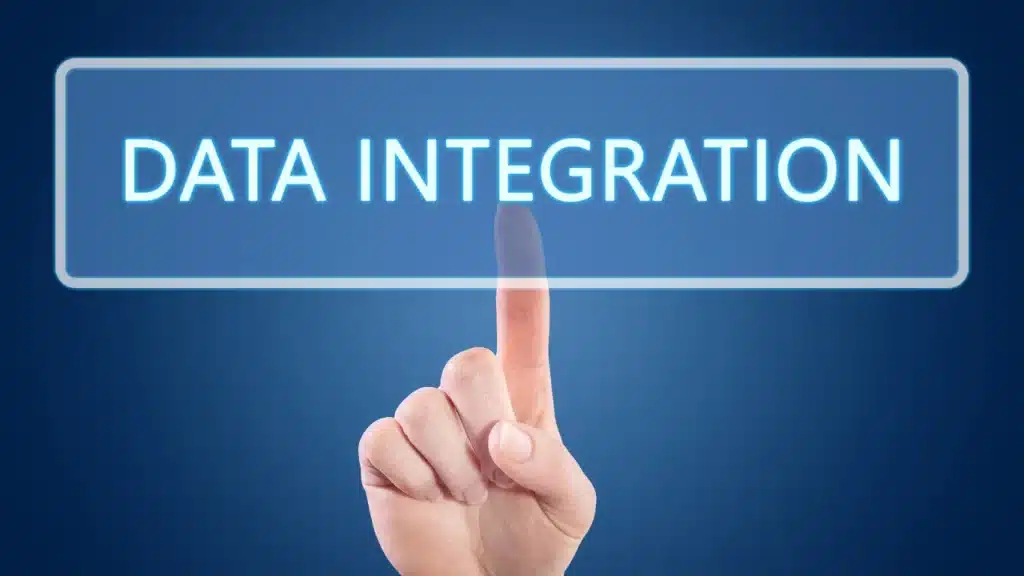
- Invest in data integration and migration technologies: Implement solutions that enable seamless data integration from various sources and facilitate data migration when required.
- Adopt secure data storage solutions: Choose appropriate technologies based on data volume, accessibility requirements, and security considerations. Implement access controls, encryption, and backup solutions to protect data.
- Leverage data analytics and visualization: Use advanced analytics techniques and data visualization tools to derive actionable insights from data. Make data-driven decisions and identify opportunities for business growth.
- Train and educate employees: Provide training and education on data management best practices. Foster a culture of data awareness and accountability within the organization.
Following these best practices, organizations can establish a solid foundation for effective data management. This, in turn, enables them to harness the power of data to drive business success and stay ahead in today’s competitive landscape.
Tools and technologies for data management
Data management involves various tools and technologies that facilitate data collection, organization, analysis, and storage. These tools and technologies enable organizations to streamline data management processes and derive insights from their data assets.
- Data management platforms (DMPs): DMPs are software platforms enabling organizations to collect, organize, and analyze large volumes of data. They provide a centralized repository for data storage and facilitate data integration and analytics.
- Data integration tools: Data integration tools enable organizations to combine data from various sources into a unified view. These tools automate the extraction, transformation, and loading (ETL) processes required for data integration.
- Data quality tools: Data quality tools assess and improve the accuracy, completeness, consistency, and timeliness of data. They provide functionalities such as data profiling, cleansing, and validation.
- Data storage solutions: Data storage solutions include traditional on-premises data centres and cloud-based storage options. Cloud storage offers scalability, flexibility, and cost-efficiency, while on-premises solutions provide more control over data.
- Data security solutions: Data security solutions protect data from unauthorized access, loss, or corruption. These solutions include access controls, encryption, and backup solutions.
- Data analytics platforms: Organisations can use data analytics tools to analyse vast amounts of data and generate insights. These systems employ advanced analytics like machine learning and artificial intelligence to find patterns and trends.
- Data visualization tools: Data visualisation tools, such as charts, graphs, and dashboards, show data in a visual style. These tools essentially allow users to analyse data and acquire insights quickly.
The demands and requirements of each company determine the tools and technology used. Evaluating and selecting solutions that align with the organization’s data management strategy and objectives is essential.
Challenges and solutions in data management
While effective data management is crucial for organizations, it comes with challenges. These challenges include –
- Data complexity: Organizations deal with vast amounts of structured and unstructured data from various sources. Managing and integrating diverse data sets can be complex and time-consuming.
- Solution: Implement data integration tools and technologies that aid in automating the process of extracting, transforming, and loading data. Leverage data management platforms to centralize data storage and facilitate data integration.
- Data quality issues: Data quality problems, such as inaccuracies, inconsistencies, and missing values, can hinder decision-making and operational efficiency.
- Solution: Implement data quality tools and practices to assess and improve data quality. Establish data governance frameworks that define data quality standards and responsibilities.
- Data security risks: With the increasing frequency of data breaches and privacy concerns, organizations must prioritize data security. Protecting data from unauthorized access is a hard challenge.
- Solution: Implement robust data security solutions, including access controls, encryption, and backup strategies. Comply with relevant data protection regulations and standards.
- Lack of data governance: With proper data governance frameworks in place, organizations may be able to establish data management policies, procedures, and responsibilities.
- Solution: Develop data governance frameworks that define roles, responsibilities, and processes for data management. Establish data governance committees to oversee data-related activities.
- Legacy systems and data migration: Upgrading or adopting new technologies often requires data migration. Data migration projects can be complex and prone to data loss or corruption.
- Solution: Plan data migration projects carefully, including data mapping, validation, and testing. Leverage data migration tools and technologies to automate the process and minimize risks.
By being aware of these challenges and implementing appropriate solutions, organizations can overcome data management obstacles and effectively handle their data assets.
The future of data management
Data management is continuously evolving, driven by technological advances and the increasing importance of data in decision-making. Several trends are shaping the future of data management –
The proliferation of big data and the advancements in artificial intelligence (AI) are transforming data management. Organizations leverage big data analytics and AI technologies to uncover insights and automate data management processes.
Cloud computing offers scalability, flexibility, and cost-efficiency, making it an attractive option for data storage and processing. Organizations are increasingly adopting cloud-based data management solutions to leverage these benefits.
With regulations like the GDPR, data privacy and compliance have become critical concerns for organizations. Data management practices must align with these regulations to protect customer data and avoid penalties. Data governance frameworks are becoming more mature, focusing on data stewardship. Organizations are assigning dedicated data stewards responsible for data quality, security, and compliance.
Organizations empower business users to access and analyze data without relying on IT departments. Self-service analytics tools enable users to explore data and derive insights independently. The future of data management lies in leveraging emerging technologies and adopting agile practices. Organizations must be prepared to embrace these changes and adapt their data management strategies accordingly.
FAQ
What is the meaning of data management?
Data management is the process of collecting, organizing, protecting, and storing an organization’s data securely, efficiently, and cost-effectively. It involves ingesting, storing, managing, and maintaining data within an organization to utilise the data for analysis and informed decision-making.
What is data management and examples?
Data management encompasses various activities that ensure data is accurate, consistent, and accessible. Some examples of data management in action include data governance, integration, and security.
What are the four types of data management?
Data management can be categorized into four main types, each focusing on different aspects of the data lifecycle:
Data Governance, Data Integration, Data Quality and Data Security.
What is the role of data management?
Effective data management enables organizations to make informed decisions based on accurate and reliable data. Additionally, it streamlines processes and improves operational efficiency. Organizations can eliminate data silos and gain a comprehensive view of their operations by integrating data from various sources. Data management is essential for ensuring compliance with regulatory requirements and mitigating risks associated with data breaches or loss. Lastly, data management provides organizations with a solid foundation for growth and innovation.
Conclusion: Data Management
In today’s data-driven world, effective data management for organizations is essential in order to stay competitive and make the proper decisions. Businesses can establish robust data management practices by understanding the critical components of data management, such as data governance, data quality, data integration, and data storage. Leveraging tools and technologies for data management, addressing challenges, and embracing emerging trends will pave the way for successful data management strategies. By mastering data management essentials, organizations can unlock the full potential of data assets and drive business growth and innovation.